From phishing attempts and malicious attachments, to fraudulent messages that look like the real deal –email scams have become a persistent threat.
For most of us as the average user, the solution seems simple: click a button to report a suspicious email, and it disappears. But for government agencies that often receive a large number of emails from the public, the challenge is much more complex, says Mr Jeremy Lee, Product Lead at Assurity Trusted Solutions, a wholly-owned subsidiary of the Government Technology Agency (GovTech).
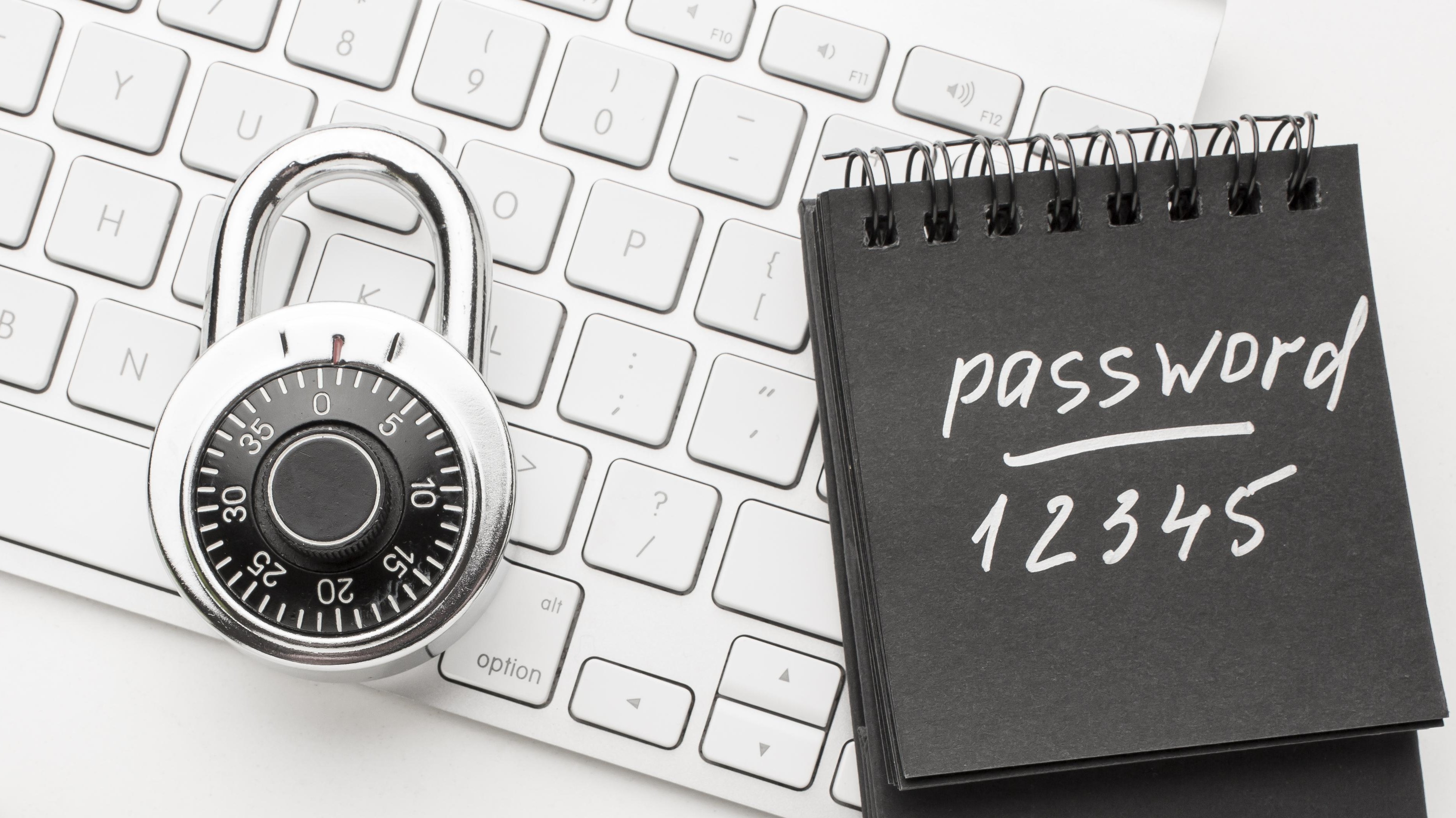
Public institutions like tax authorities and ministries are flooded with emails from citizens every day, and “it’s hard to immediately determine whether they are malicious or harmless”, Mr Lee explains. To address this, employees are encouraged to report suspicious emails, which are then evaluated by the cybersecurity teams.
The scale of the problem is staggering. Mr Lee shares that behind the scenes, a small team of cybersecurity professionals has to sift through about 10,000 emails a month to determine which ones are genuinely dangerous. This sheer volume makes it unsustainable, leading the team to explore technological solutions.
Playing catch up
Initially, the team relied on machine learning (ML) models, including deep learning, to combat email scams. “(They were) effective to a certain extent,” says Mr Lee.
A significant limitation: These models require deep technical expertise to set up and maintain. They also required constant retraining as new threats emerge. “It’s a very narrow form of AI because it can only tackle specific types of malicious emails,” Mr Lee explains, highlighting the need for more adaptive and scalable solutions.
There’s another challenge; as email scams evolved, so did the complexity of the threat. Emails sent by scammers now look more polished, as they start using generative AI tools like ChatGPT. Previously, phishing emails often contained obvious red flags such as spelling mistakes or clunky phrasing, but that’s no longer the case. “Now, phishing emails are perfect, sometimes even better than a human could write,” Mr Lee says. This shift has forced cybersecurity teams into a constant cycle of retraining models to keep up with these increasingly polished attacks.
And this is where generative AI comes into the picture. The team at GovTech saw an opportunity to leverage generative AI to stay ahead of the scammers. Unlike traditional AI, which requires constant manual retraining, generative AI models can adapt more fluidly to new types of scams, Mr Lee says.
Beyond just identifying threats, generative AI offers another key advantage - Explainability in Machine Learning (ML). One of the longstanding challenges with traditional ML models is the “black box” nature of their decision-making. Users would often question why an email was labeled as malicious, but the model would only provide a score without much clarity.
Powered by Large Language Models (LLMs), generative AI can offer more detailed, human-readable explanations, which helps educate users about why certain emails are flagged as dangerous. “This makes the system more transparent, so users can understand the reasons behind each decision,” Mr Lee explains.
The power of language models
Selecting the right LLM to combat email scams is no easy task, especially with so many options available in the market now.
“We take a more product-focused approach to find the best fit for our needs,” Mr Lee says. Instead of diving straight into technical aspects, his team looked at each model’s real-world performance and how well it aligns with their goals. This makes the decision process more practical and user-centric than purely technical.
“We would compare models based on their strengths and weaknesses,” explains Mr Lee. One key tool in their evaluation process is benchmarking. Models like Lama, Gemini, and other newer entrants are measured against standardised benchmarks, such as the General Language Understanding Evaluation (GLUE) framework. “GLUE essentially helps us understand how well a model can comprehend the problem statement and how robust its responses are,” he adds.
The key advantage of LLMs is their ability to adapt to emerging threats much faster than traditional models.
Mr Lee notes that traditional machine learning models often require about a month to gather and retrain on new data. By then, phishing tactics may have already shifted. In contrast, LLMs can quickly identify new trends and retrain themselves. “Recently, we saw a spike in phishing attempts using fake Grab invoices,” Mr Lee shares. “We gave the LLM model examples of these new threats and were able to deploy an updated version within just a few days.”
It’s all about being agile, he emphasises. And in today’s fast-moving digital landscape, that agility is crucial to protecting users from increasingly sophisticated email scam tactics.
For more information on NUS-ISS Executive Education Programme in Gen AI visit here
For more information on NUS-ISS Blended Learning Programme in AI and Cybersecurity visit here.